How Does Entity Extraction Work?
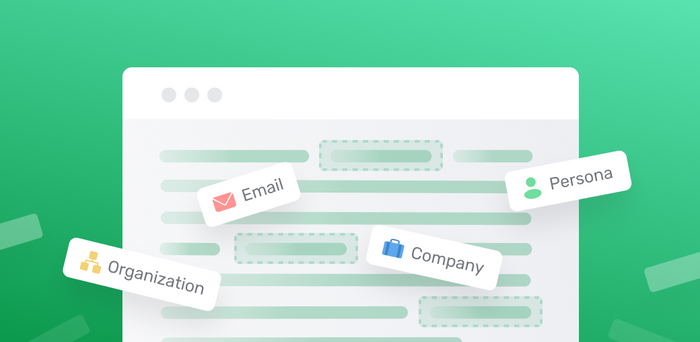
Information can be extracted from text using the technique of “entity extraction,” also known as “entity name extraction” or “named entity recognition.” A machine-readable (or structured) version of unstructured data is made available for typical processing tasks such as obtaining information, identifying facts and answering inquiries. So how does it all work in the end?
The Importance of Entity Extraction
Unstructured data can be found in a variety of formats, including documents, spreadsheets, websites, and social media. You can use the information in documents to your advantage if you can recognise the various types of entities they contain, such as people, places, organisations, concepts, numerical expressions (such as dates, times, phone numbers, currency amounts, etc.), and temporal expressions (such as dates, frequency, times, duration, etc.).
Analysis and investigative journalism are two examples of situations where people may not know what the information includes and what they should be looking for when they first get their hands-on terabytes of data.
To have a better understanding of unknown data sets, entity extraction can show at a minimum who and what the data focuses on. All the names of people, companies and brands may now be viewed in an (organised) corpus that can be used as a starting point for further research and analysis.
Workplace Entity Extraction
To effectively identify and classify items, extraction methods must overcome a number of language difficulties. While humans can easily discern between different types of names (e.g., people, places, organisations, products, etc.), the ambiguities of language make this a particularly difficult task for robots to accomplish.
When relying solely on keywords, a system can’t tell the difference between a word’s several possible connotations or even its context. In the case of the word “orange,” there is no way to tell whether it refers to the colour, a fruit, a region, or a business.
Pattern matching, syntax, linguistics, semantics or any combination of these approaches can be used to extract entities from a piece of text. Entity extraction based on semantic technologies enables a wide range of relevant downstream operations that are beneficial to a wide range of corporate processes in various industries, including marketing, sales, and customer service. Among them are:
Extraction of Entity Relationships:
As well as revealing intricate linkages through indirect connections, it reveals direct interactions, connections, or occurrences between separate things.
Linking:
Links knowledge repositories together There are several ways in which a corpus can be linked to a location on a map or entities can be linked to other sources of information
Extraction of the facts:
Provides a specific response to a query by retrieving all of the data associated with an entity from a corpus. This goes beyond simply providing a list of “answers” to a question.
These three processing steps form a strong foundation for transforming business processes across a company. However, their effectiveness is contingent upon knowledge, which can only be obtained through a semantic method. You can rely on entity extraction to do the heavy lifting for you. Why not let us show you how it’s done.